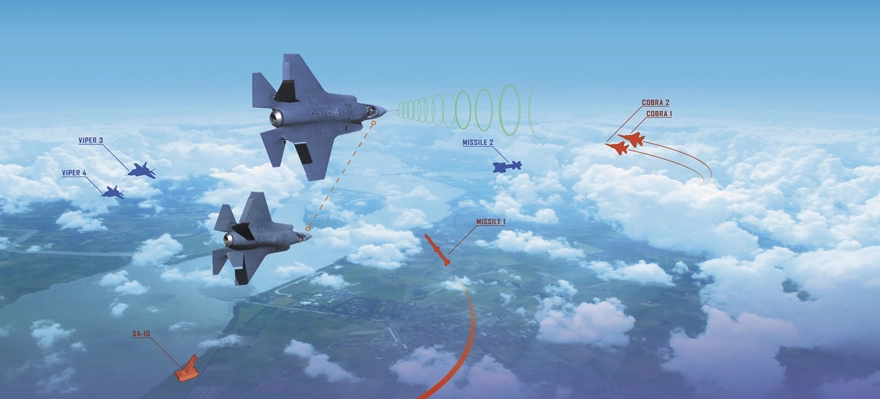
Master Thesis on Reinforcement Learning for Combat Aircraft
Research in autonomous combat aircraft decision-making has shifted from rulebased systems towards deep reinforcement learning. Although reinforcement learning has shown great promise in other technically difficult domains, not many successful applications within the combat aircraft domain exist. Solutions often focus on control of the aircraft, rather than applying it to strategy and cooperation. Furthermore, few case studies on implementation exist, and effective solutions often require many resources, standing in the way of implementation.
This thesis explores the effectiveness of reinforcement learning in the combat aircraft domain through an extensive literature review and implementation. It develops a novel solution focusing on cooperation and strategic planning with limited resources, rather than the direct control solutions that is common in the current body of work. A case study for reinforcement learning in Airbus’ environment has revealed fundamental challenges in the domain that have not been previously investigated, and serves as a model for further developing such a solution into a realistic prototype.
Based on the proofofconcept and literature review, recommendations are given to the stakeholder Airbus Defense and Space on how such a solution can contribute to the Embedded Combat Aircraft Training System (ECATS).